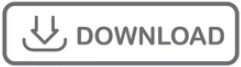
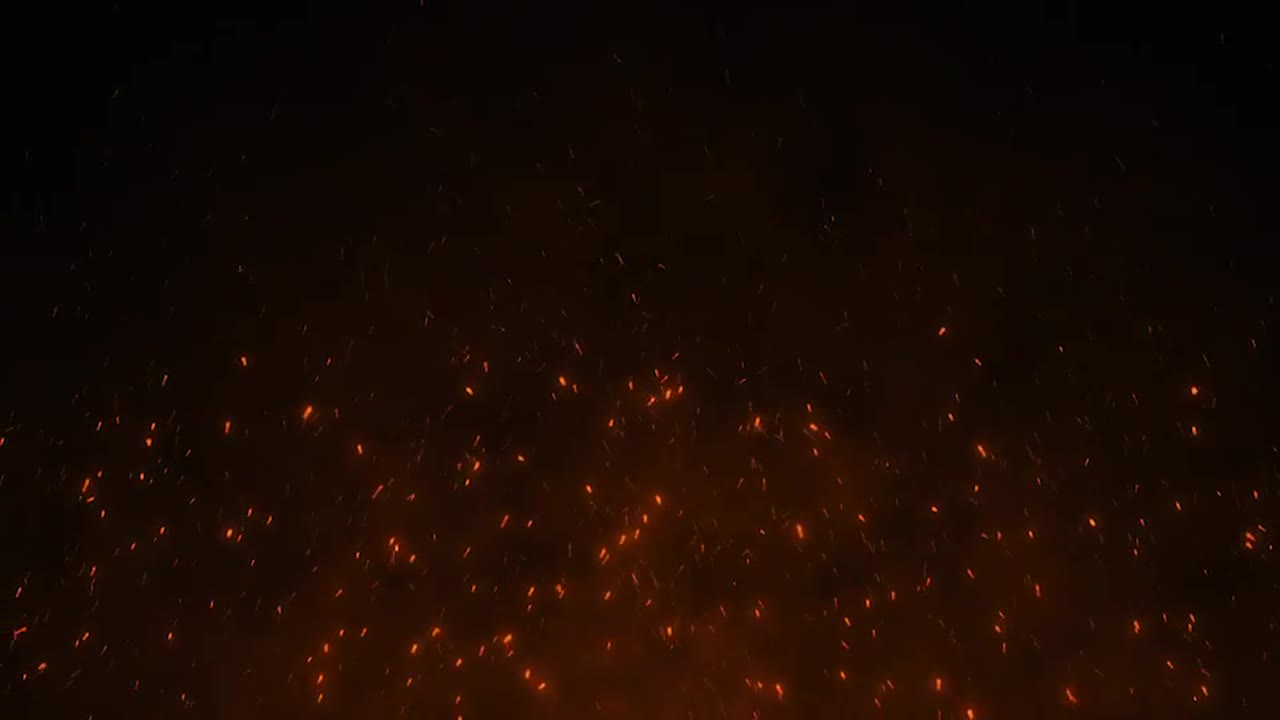
Due to the limited coverage area, conventional sensors are not efficient in large rooms and open spaces like backyards, car parks, forests, etc. This gives plenty of room for a normal fire to spread rapidly. A fire has to burn for a while to reach the sensor and to exceed the set threshold point to initiate a warning alarm. The commonly used point sensors like smoke, heat, and gas sensors have limited capabilities. There are various fire sensing technologies developed to reduce fire accidents. With the increased complexity in building operations, it is important to improve sensitivity and accuracy of fire detection. According to one of the common building communication standards BACnet, fire safety is given the highest priority in building automation.
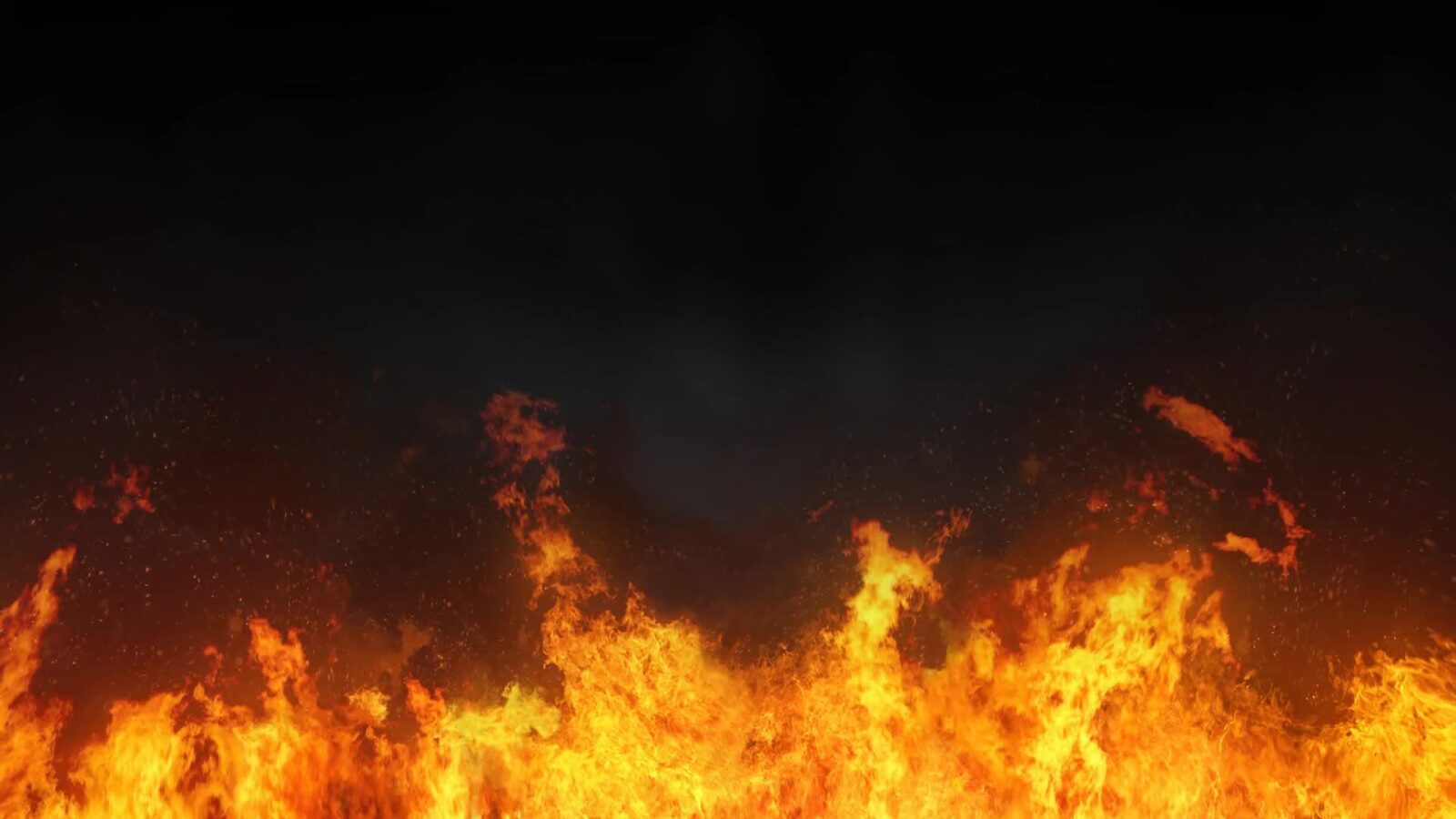
Fire growth and the maximum heat release rates mainly depend on the building design features including flammable cladding and the types of combustibles (fire loads) such as interior decorations, furniture, etc.
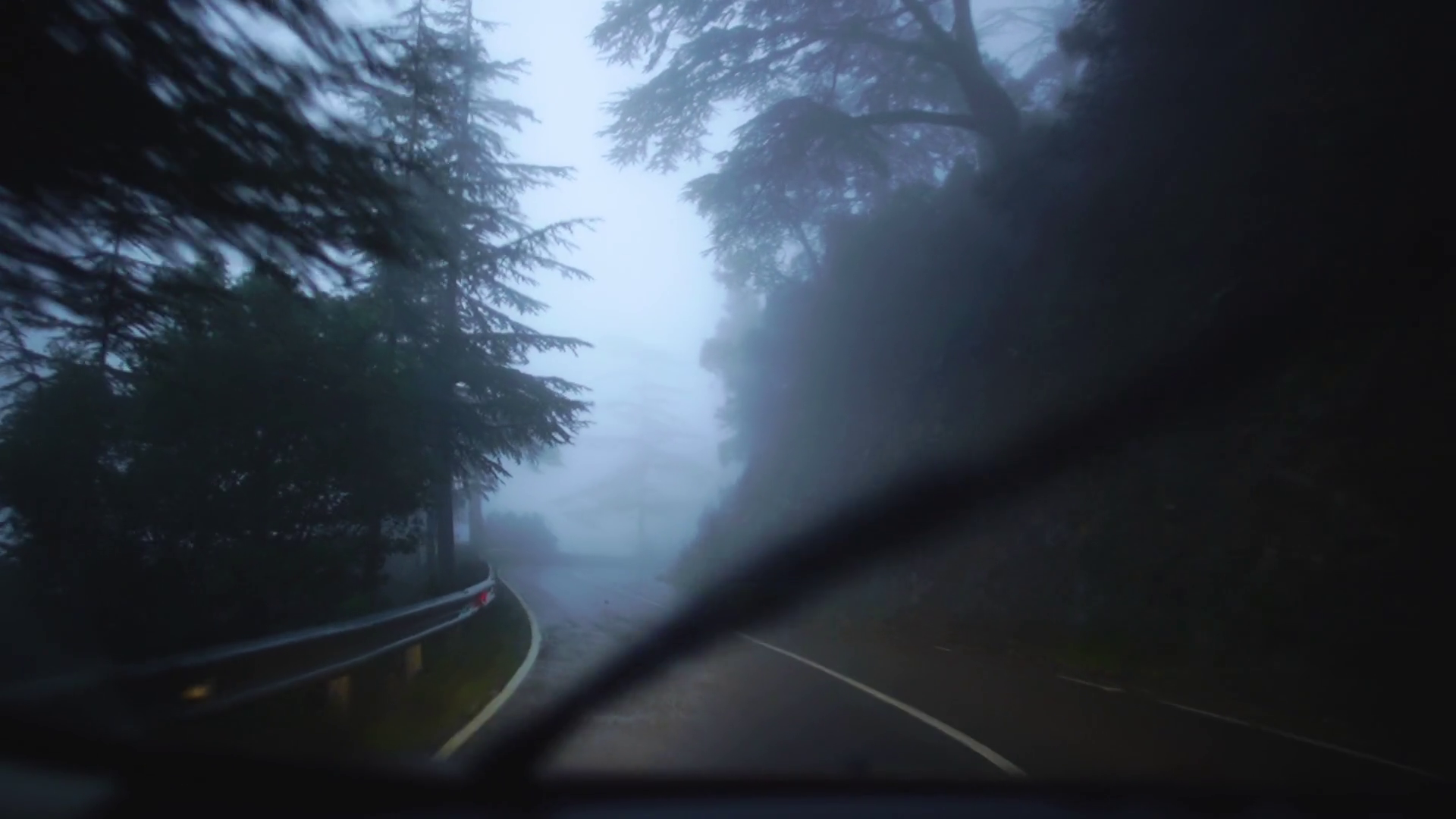
The stages of a fire can be described as incipient (ignition), growth, fully developed, and decay. Fires happen due to various reasons such as negligence, electronic device malfunctioning, cooking, smoking, flammable liquid and gas leakages, arson, etc. Yet, fires can be avoided or controlled if identified at the incipient stage. Experimental results for the real-time strategy which works at a speed of 12 frames-per-second show 95.34% and 92.39% fire and human motion detection accuracy levels respectively.Įarly fire detection in residential or industrial buildings is very important to take appropriate actions to prevent human and property losses, physical and mental trauma. Experimental results show that the ShuffleNet architecture yields the highest prediction accuracy of 87.8%. Various pre-trained DNN architectures are adopted to train and identify the collected data for background (no motion), human motion, and fire categories: small quasi-static and spreading fires. The corresponding wavelet coefficients are converted into RGB spectrum images that are then used as inputs for a deep convolutional neural network. These signals are processed using one-dimensional continuous wavelet transform to perform feature extraction. Since the PIR sensor is sensitive to sudden body motions and emits a continuous time-varying signal, experiments are carried out to collect human and fire motions using a PIR sensor. This paper presents a differential pyro-electric infrared (PIR) sensor and deep neural networks (DNNs) based method to detect fire in real-time. Early detection of fire is key to mitigate fire related damages.
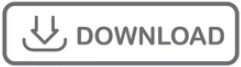